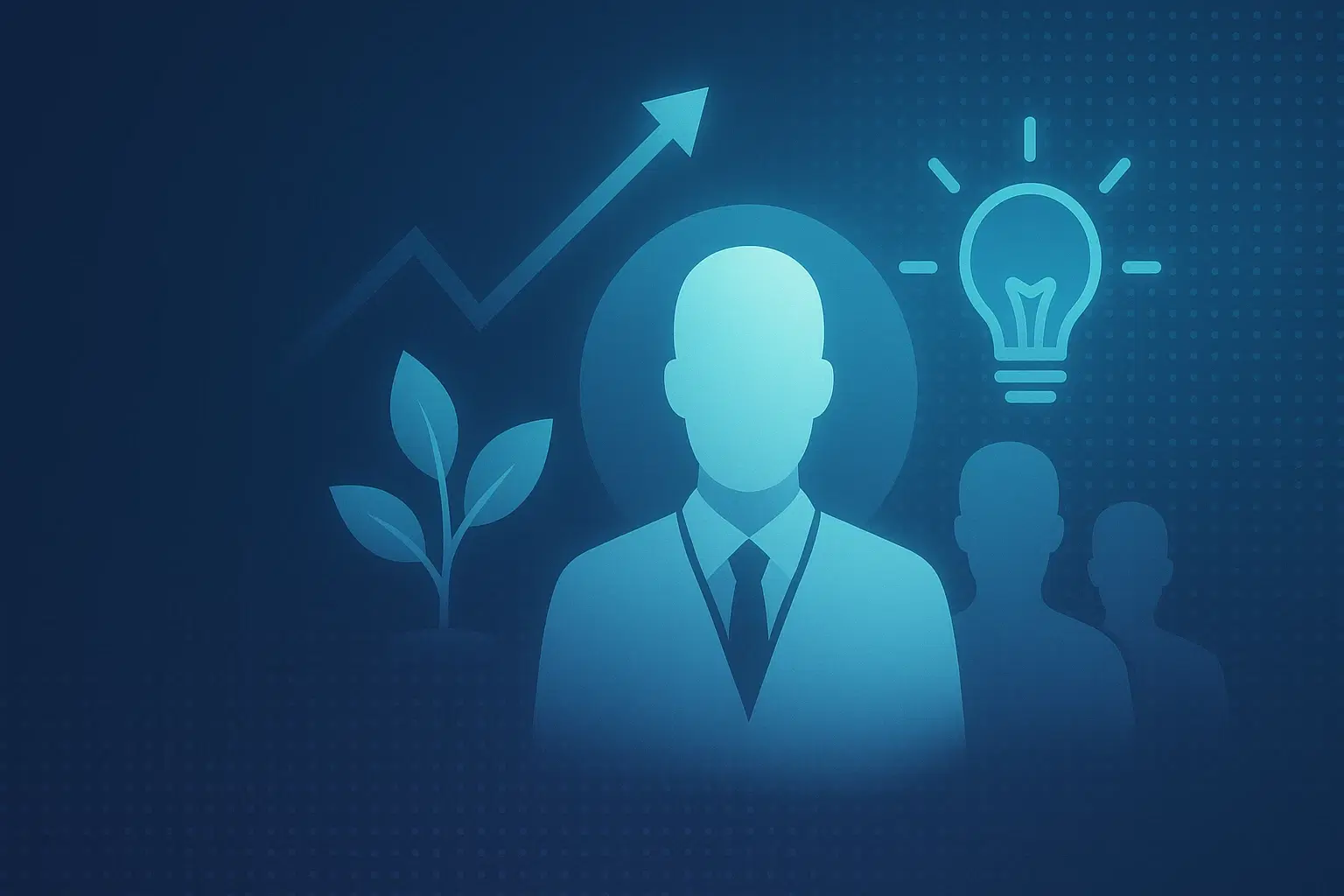
AI Use Cases & RoI
•02 min read
The integration of Generative AI into the enterprise is not just a technological shift; it's a profound transformation that impacts the workforce at every level. This seventh blog in our series focuses on the critical human capital strategies required to navigate this evolution, including cultivating the necessary talent, leading organizational change effectively, and ultimately enabling greater productivity through human-AI collaboration.
The AI talent landscape presents a significant challenge for many enterprises. There is a persistent skills gap, with high demand for specialized roles such as data scientists, ML engineers, prompt engineers, and AI governance experts. Competition for this talent is fierce, and hiring can be costly and time-consuming.
Addressing this requires a multi-pronged approach to workforce adaptation:
A key principle is empowering the workforce by providing access to GenAI tools for domain experts. The concept of "Get AI in the hands of experts" recognizes that individuals with deep domain knowledge are best positioned to identify high-value use cases and leverage AI to solve specific business problems. Providing them with user-friendly AI tools and platforms can unlock significant innovation.
Finally, developer enablement is critical for accelerating the development and deployment of GenAI applications:
Using AI Coding Assistants (e.g., GitHub Copilot): Leveraging AI-powered tools to automate code generation, improve code quality, and accelerate software development workflows.
Internal Platforms: Developing internal platforms (like Mercado Libre's Verdi) that streamline the AI development lifecycle, providing developers with easy access to models, data, and deployment tools.
Streamlined MLOps: Implementing efficient MLOps practices to automate the deployment, monitoring, and management of AI models, freeing up data scientists and ML engineers to focus on innovation.
Human capital is a critical enabler of successful GenAI adoption. By proactively cultivating talent through reskilling and targeted hiring, leading change effectively through clear communication and supportive policies, and empowering the workforce with access to AI tools, enterprises can unlock new levels of productivity and innovation. Investing in human capital in the GenAI era is not just about filling skills gaps; it's about fostering a culture of collaboration and continuous learning that will drive long-term success. Our final blog in this series will explore the future of GenAI, navigating advanced applications and anticipating future disruptions.
Comprehensive Reskilling and Upskilling Programs: Investing in training programs to equip existing employees with foundational data literacy, proficiency in AI tools, and the critical thinking skills needed to work effectively alongside AI. This includes training for domain experts to become "citizen AI developers" who can leverage GenAI within their specific areas.
Targeted Hiring Strategies: Identifying critical skill gaps and developing strategic hiring plans to attract and retain specialized AI talent. This may involve offering competitive compensation packages, fostering a culture of innovation, and providing opportunities for professional growth. 1
Focus on Prompt Engineering: Recognizing the emerging importance of prompt engineering as a crucial skill for effectively interacting with and guiding large language models. Investing in training and developing expertise in this area is essential.
Effective change management practices are crucial for a smooth transition to an AI-augmented workplace:
Strong Leadership Communication: Clearly articulating the vision for AI adoption, addressing employee anxieties about job security, and emphasizing the opportunities for augmentation and new roles.
Establishing Clear Usage Policies: Developing guidelines and best practices for using GenAI tools responsibly and ethically, ensuring data security and compliance.
Promoting a Culture of Experimentation and Human-AI Collaboration: Encouraging employees to explore the potential of GenAI tools and fostering a collaborative environment where humans and AI work together to achieve better outcomes.